Summary
The SIRENE (Satellite Information for Resilience Monitoring and Early warning of Ecosystem tipping points) project aims to enhance understanding of terrestrial ecosystems and in particular the detection and prediction of their climate-driven tipping points.
The project is developing improved and uncertainty-aware methods for resilience monitoring. In SIRENE, we will use combine AI-driven analyses with advanced Earth Observation (EO) techniques and will benchmark results with ground data - such as CO2 fluxes or tree diameter. With a focus on vulnerable regions like tropical rainforests, boreal forests, and drylands, this will help to identify early warning signals of potential ecosystem collapse.
The ultimate goal is to create a robust framework for tracking tipping points, supporting climate risk assessments, and informing global reports like those from the IPCC and IPBES.
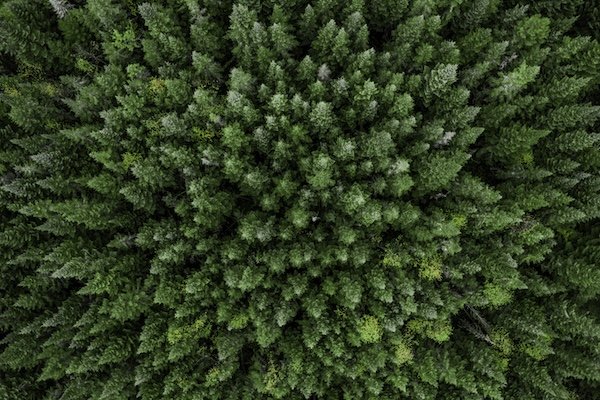
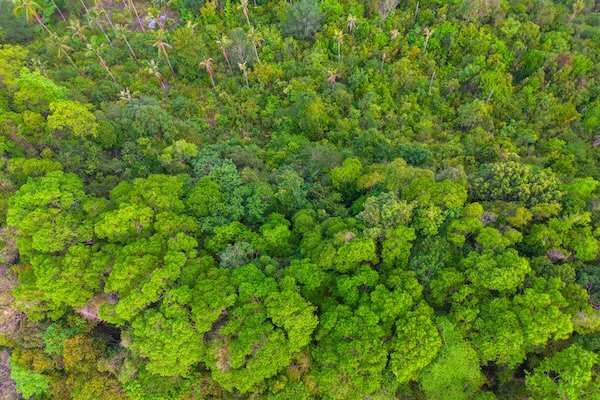
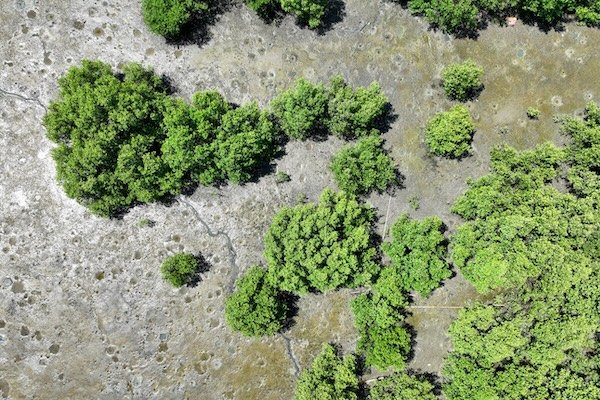
Background
From a policy perspective, the lack of reliable early warning systems prevents timely intervention. Due to uncertainties in climate risk assessments, reports from organizations like the IPCC and IPBES may underestimate ecosystem vulnerability. Furthermore, there still exists a disconnect between science and policy, as complex resilience indicators are difficult to translate into actionable measures.
In particular, a critical challenge is the uncertainty in defining intervention thresholds. Since tipping elements develop gradually before collapse, policymakers struggle to determine when action is necessary to prevent irreversible ecosystem loss. Additionally, regional variations in climate impacts mean that policies must be localized rather than generalised. Moreover, few best-practices exist, making the standardization of resilience metrics difficult and thus, hindering the comparison of resilience results.
One major scientific challenge is the complexity and nonlinearity of tipping points, which make them not only difficult to predict but sometimes irreversible. Current Earth system models (ESMs) struggle to incorporate abrupt ecological changes such as large-scale tree mortality, fire regimes, and ecosystem shifts. Additionally, data uncertainty remains a challenge, as satellite observations are affected by sensor biases, unrelated environmental conditions such as cloud cover, and variations in data processing methods (e.g., cloud correction, vegetation indices). This introduces high uncertainty to estimates of resilience and resilience changes.
Statistical limitations further complicate predictions. Metrics like Critical Slowing Down (CSD), used to identify early warning signals, may be biased by measurement noise or external influences. Additionally, ecosystems respond differently to stressors such as drought, fire, and land-use change, making it difficult to generalize resilience indicators. Lastly, while AI and machine learning could enhance monitoring, their potential remains largely unexploited in ecosystem resilience studies.
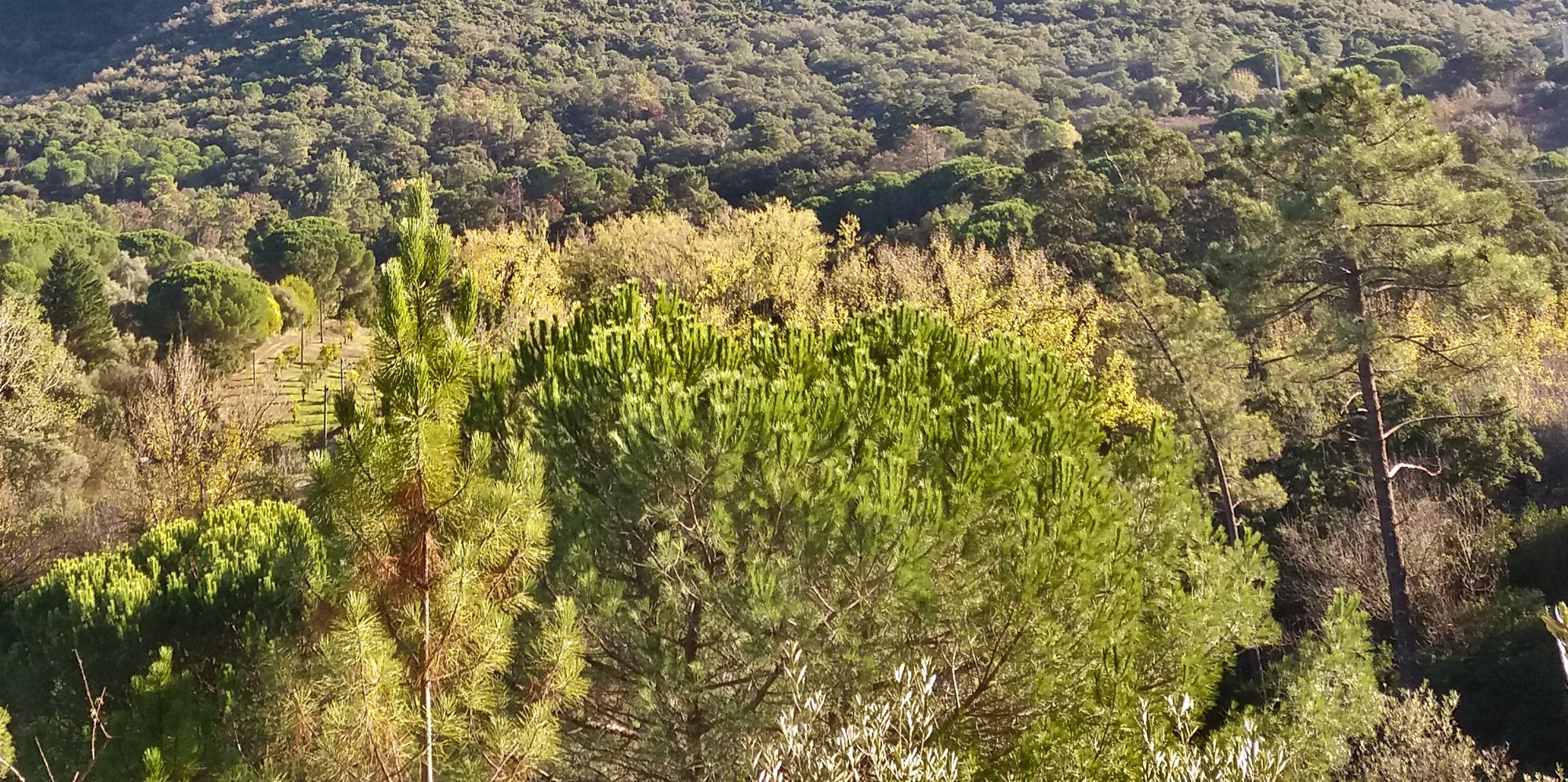
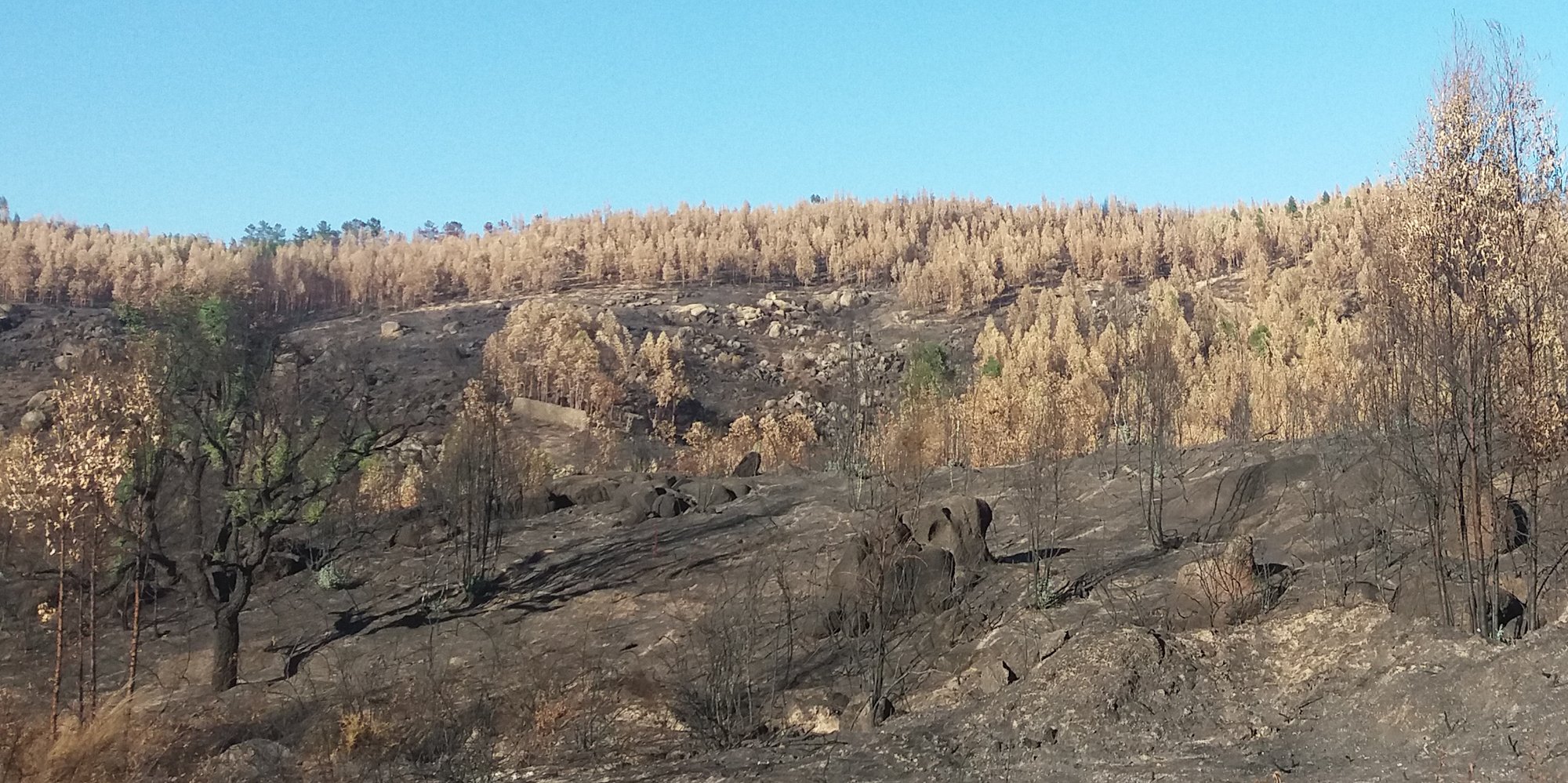
Aims and objectives
The SIRENE project aims to enhance the detection and prediction of climate-driven tipping points in terrestrial ecosystems by developing more reliable resilience metrics and improving early warning systems.
One major focus is improving ecosystem resilience assessments. Current resilience metrics, such as those based on Critical Slowing Down (CSD), are limited by the noise, short time-series data, and external influences. This project seeks to refine these resilience estimation of terrestrial ecosystems by incorporating multi-sensor satellite data, enhanced and validated with ground-based data, long-term climate records, and robust resilience estimators. We will employ machine learning for detecting patterns and improving prediction accuracy. The further integration of AI-driven analysis and Earth system modeling will provide a more holistic understanding of ecosystem resilience.
To make the results more relevant for policymakers, we will quantify and propagate uncertainties from data and resilience estimation into the final results. This will allow clearer risk assessments. With this, the SIRENE project enables more effective climate adaptation strategies, ecosystem conservation efforts, and land-use planning decisions. We also seek to develop region-specific resilience assessments, recognizing that ecosystems respond differently to climate stressors such as drought, fire, and deforestation. Understanding how different types of ecosystems respond to climate extremes will help guide restoration efforts and improve ecosystem management.
The SIRENE project envisions a future where climate-driven tipping points can be predicted with greater certainty, allowing for timely policy interventions and conservation actions. Furthermore, by standardizing resilience metrics and ensuring they are accessible to policymakers, the project aims to foster stronger science-policy collaboration. Ultimately, this research will contribute to global efforts to mitigate climate change impacts and protect vulnerable and valuable ecosystems.
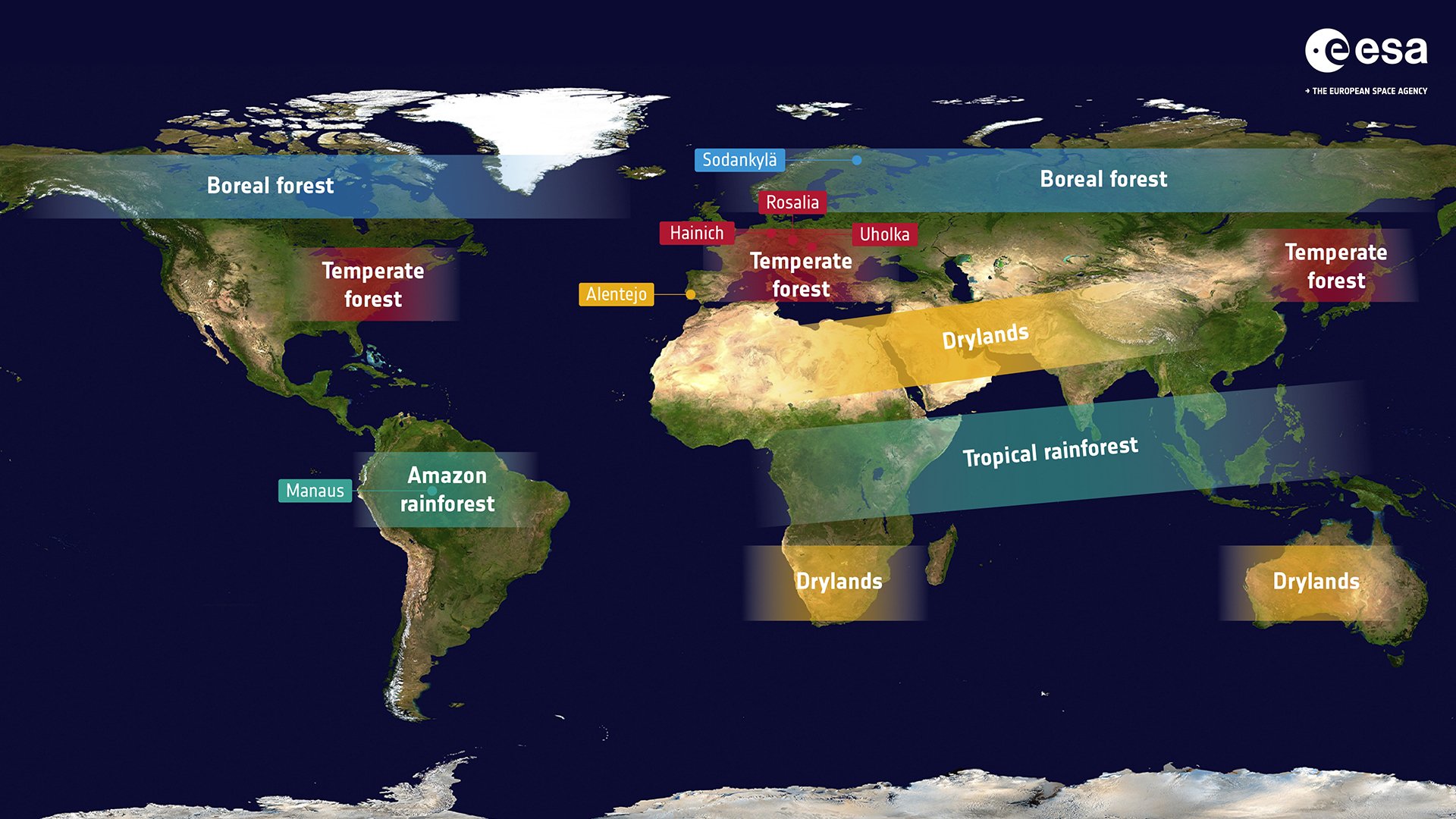
Project plans
Understanding resilience is crucial for predicting vegetation ecosystems’ responses to environmental changes. The SIRENE project aims to improve resilience quantification by integrating Earth Observation (EO) and ground-based data, refining metrics, and addressing uncertainties. This includes the following steps.
Advancing Methods for Resilience Monitoring
To enhance resilience monitoring, we will evaluate the quality of a broad range of datasets, focusing on uncertainty assessment and fitness-for-purpose. Our analysis will determine whether dataset uncertainties are adequately represented. Furthermore, we will advance resilience metrics by improving Critical Slowing Down (CSD)-based resilience metrics, e.g. by accounting for state-dependent and time-dependent changes in vegetation.
Benchmarking and Model-Based Assessment
Resilience metrics will be benchmarked against empirical recovery rates from disturbances like droughts and fires, in satellite but also ground-based data. Simulations of process-based models in controlled environments help us understand the expected metric consistency. Furthermore, we will employ Machine Learning to determine the optimal resilience quantifier by integrating different datasets.
Uncertainty Quantification and Trend Analysis
A key component of our research is integrating uncertainty propagation into resilience assessments: we will develop an uncertainty-aware framework to improve future resilience studies. Employing our own framework, we will evaluate the robustness of resilience trends, and relate them to environmental factors such as temperature, precipitation, CO2 levels, and land-use changes. By addressing methodological gaps, refining resilience metrics, and incorporating uncertainty assessments, the SIRENE project will enhance the reliability of global vegetation resilience estimates and inform climate adaptation strategies.
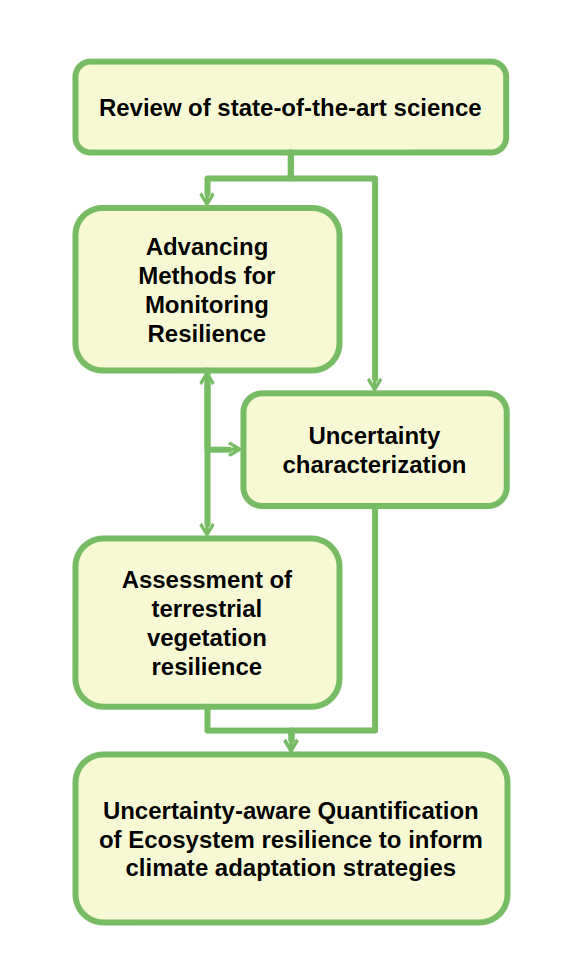
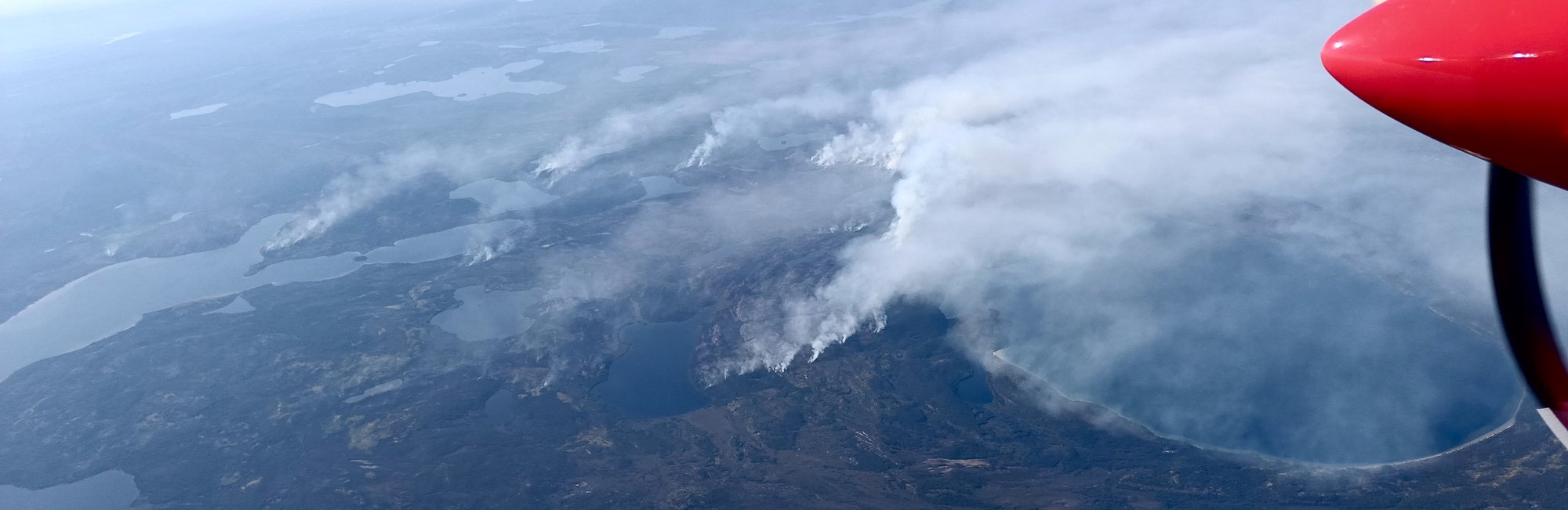
The SIRENE project is led by the Technical University of Munich (TUM) and comprises 5 contributing partner organisations:
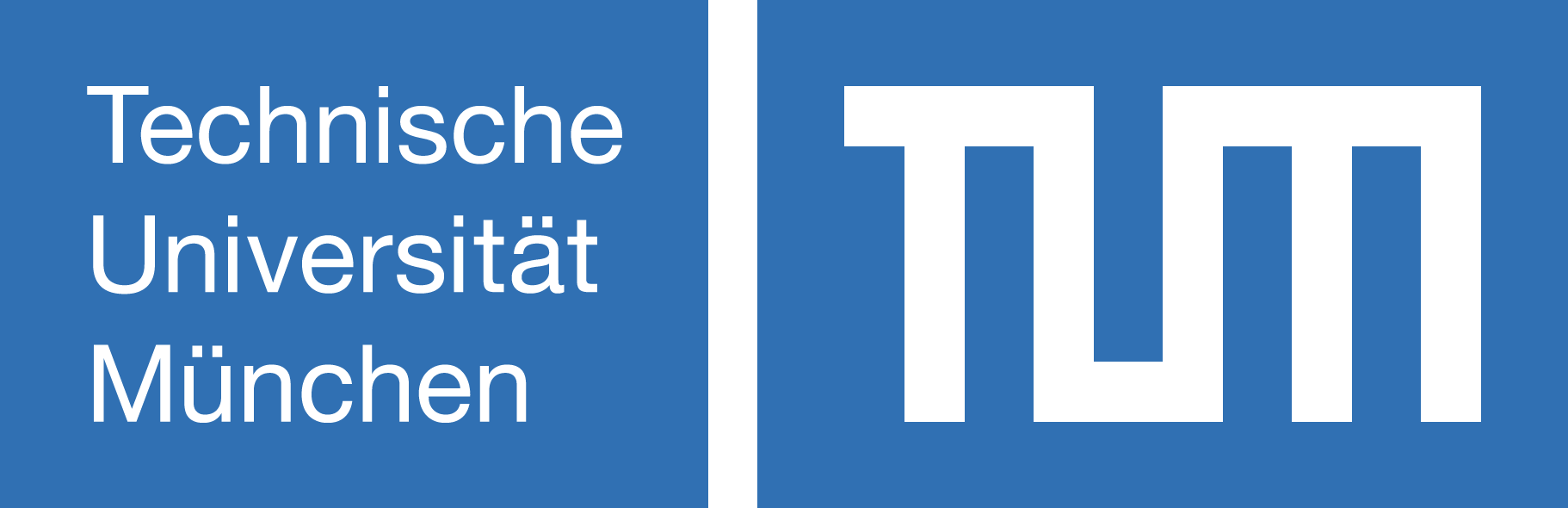
Technical University of Munich (TUM)
Project role: Prime
- Niklas Boers - Project and Task Manager
- Cornelius Senf - Co-Manager and Supervisor
- Roland Pail - Co-Manager and Supervisor
- Sebastian Bathiany - Co-Manager, Supervisor and Researcher
- Lana Blaschke - Co-Manager and Researcher
- Steve Teo - Researcher
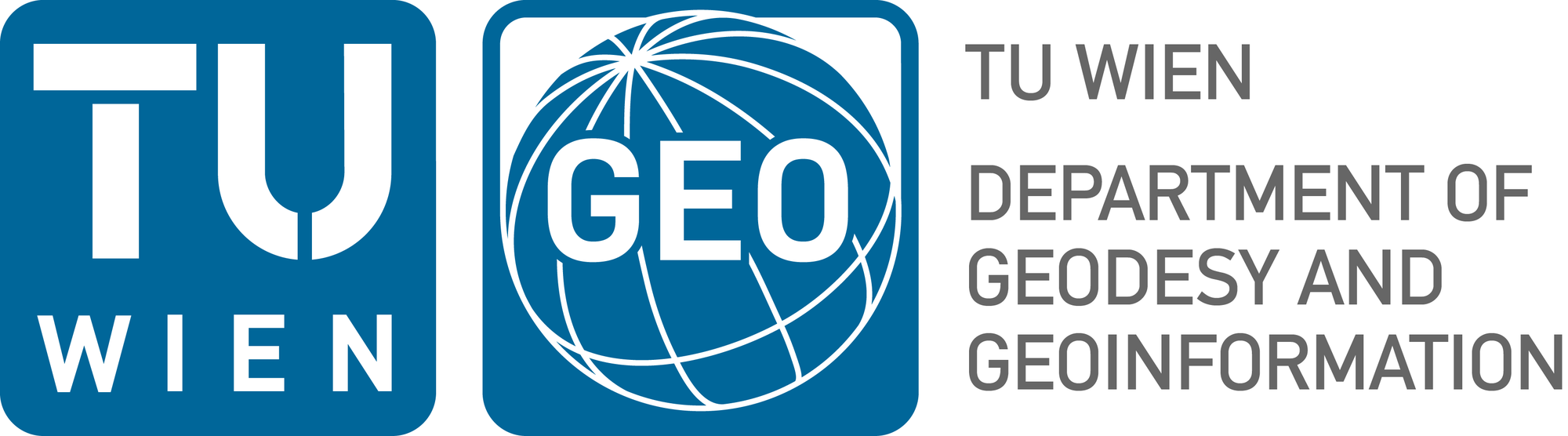
Vienna University of Technology (UTW)
Project role: Lead on dataset quality assessment and resilience monitoring
- Wouter Dorigo - Manager
- Ruxandra Zotta - Co-Manager and Researcher
- Nicolas Bader - Researcher
- Nils Voß - Researcher
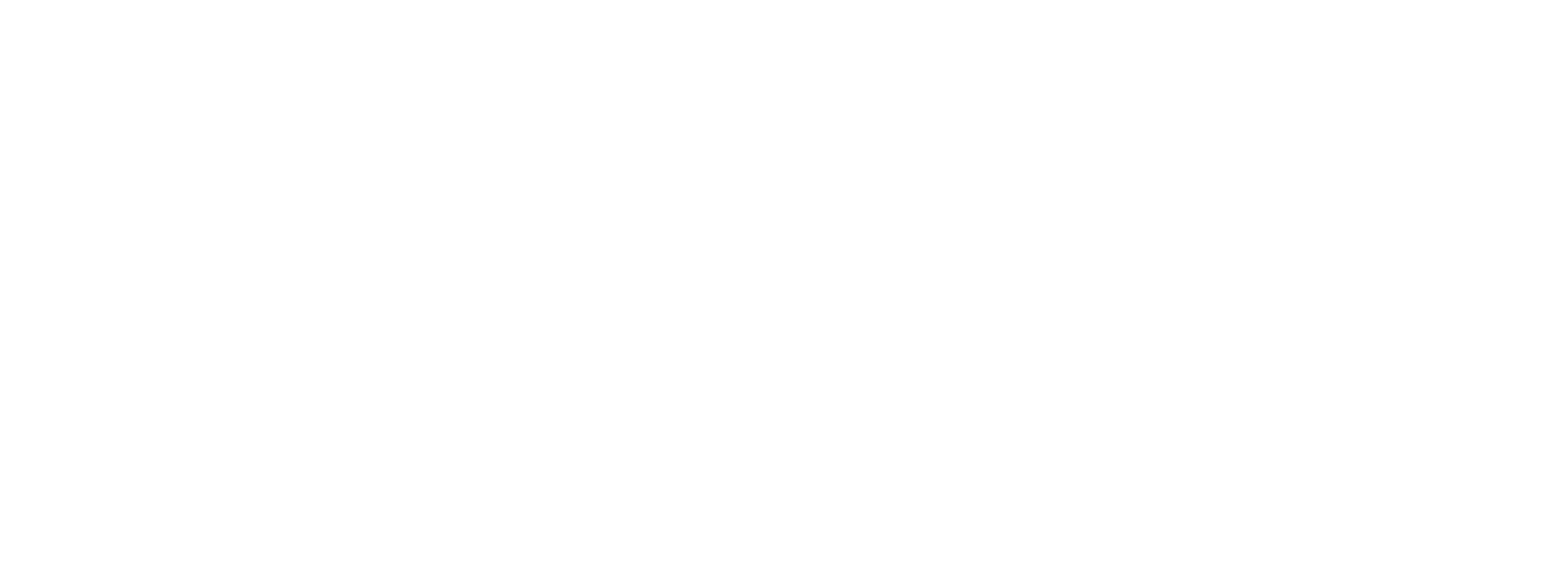
National Physical Laboratory (NPL)
Project role: Lead on uncertainty characterisation
- Bernardo Mota - Manager and Researcher
- Harry Morris - Co-Manager and Researcher
- Rasma Ormane - Researcher
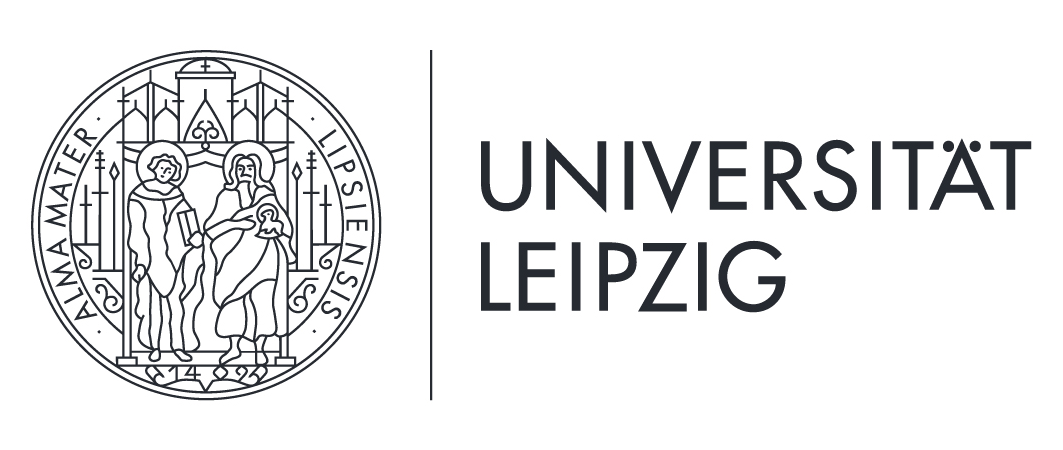
Leipzig University (ULEIP)
Project role: Lead on remote sensing and machine learning
- Ana Bastos - Co-Manager and Supervisor
- Miguel Mahecha - Co-Manager and Supervisor
- Sophie Wolf - Researcher
- Xiaona Wang - Researcher
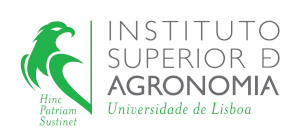
University of Lisbon, School of Agriculture (ULISB)
Project role: Lead on ecology and in-situ data
- Maria Caldeira - Co-Manager and Supervisor
- José Cardoso Pereira - Co-Manager and Supervisor
- João Silva - Co-Manager and Supervisor
Science Leader: Prof Niklas Boers, n.boers@tum.de
Project Manager: Lana Blaschke, lana.blaschke@tum.de
ESA Technical Officer: Sophie Hebden, sophie.hebden@esa.int
Latest news & events
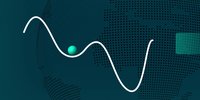
Integrating tipping points into climate science: A call for enhanced monitoring and modelling
Why tipping points in climate change matter – and what must be done
Learn more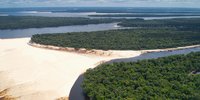
New position: Researcher in Ecosystem Resilience and Climate Data Integration
SIRENE news
Learn more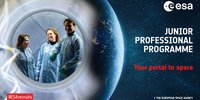
New Position: Junior Professional in AI for Climate Science
Opportunity for early-career Artificial Intelligence talent to join ESA’s Actionable Climate Information Section
Learn more